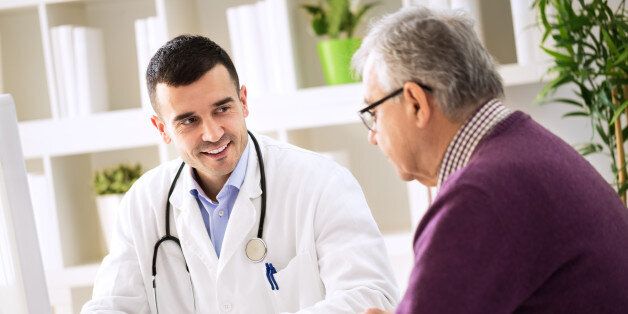
We think we know a heart attack when we see one: a high pressure situation, a hand to the chest, and the loosening of the tie. Women are, however, more likely to have a "silent heart attack" which manifests itself without pain. They are also less likely than a man to survive their first heart attack.
Whilst we might view one another as being very similar, our bodies are not. For example, people from developing countries have a higher risk of diabetes, and people with low metabolisms can process medicine slowly, causing drugs to accumulate.
Understanding ill-health is incredibly difficult. For sufferers of the same illness, there is variation in genetic and environmental factors, symptoms experienced, treatments given, and the responses to those treatments. If I were a doctor, I would sigh and wish that humans were simple machines, perhaps with a convenient data port to extract diagnostics.
Given this complexity, it might surprise you that the majority of medical research has been done on a select group of people: Caucasian men. It could be argued that the gender split makes sense: firstly, it is unethical to give a drug to women if its effects on a foetus are unknown, secondly, to test a treatment's impact effectively, it is also important for the people being studied to be as stable as possible. Thanks to hormonal fluctuations, women are ever changing, which can make it difficult for a researcher to isolate their test results.
Taking the results of a narrow study and applying it to a whole population can be risky. This was sharply underlined in 2013 when the FDA realised people were overdosing on sleeping pills containing the drug Zolpidem. Women had particularly high levels of it in their system. Why? Because they metabolised the chemical more slowly.
The drug was first tested in the mid 1980's. The fact it took over a decade to realise this is troubling, and was a result of decisions made on data that nobody realised was biased. An FDA officer said the drug came to their attention because of an accumulation of knowledge over time. That knowledge included a number of people reporting the drug caused them to crash their car.
Following this journey of a drug trial to potential car crashes is sobering, and it's only one of many examples. Medical data is just not as straightforward as we would like.
The healthcare industry is beginning to see the emergence of companies applying AI technologies to medical challenges. Programmers create algorithms which learn from large volumes of data. The algorithms then apply their knowledge to situations: Does that scan look OK? What should the doctor do next? Does this person need emergency surgery? All those learnings could have been formed from data that may not have been tested on everyone. If AI is the ultimate machine, data is the fuel and right now we're filling our space ship up at the local petrol station and hoping to reach Mars.
Just as a patient takes the dose of medicine their doctor prescribes, a person interacting with a nice shiny app is naturally going to trust what they are told. If I entered jaw ache and sudden, extreme tiredness into a GP app, would it know that as a woman these could be signs of me having a major heart attack, or would it tell me not to worry? As with the sleeping pills, if developers use biased data they'll be stress testing their software on real people.
Biases can only be fixed if you're aware of them, yet the clinicians who may have learned from past research are not the people who develop algorithms. How can we expect a programmer with a Computer Science degree to recognise that the data he is looking at is biased? Does the company behind a shiny blood pressure tracking app or medical advice portal have the budget to pay for a medical advisor?
Some companies are diligent in the way they apply technology to health. A great example is DeepMind and their close partnership with the NHS. In addition, any AI which is used in a medical setting is highly regulated.
The possibilities of applying AI to healthcare are in principle limitless. It has the potential to unpick the interrelated nuances that impact a person's health and dramatically improve lives in the future. In the meantime, let's avoid recreating the mistakes of the past and embrace the hype with an analytical mind.