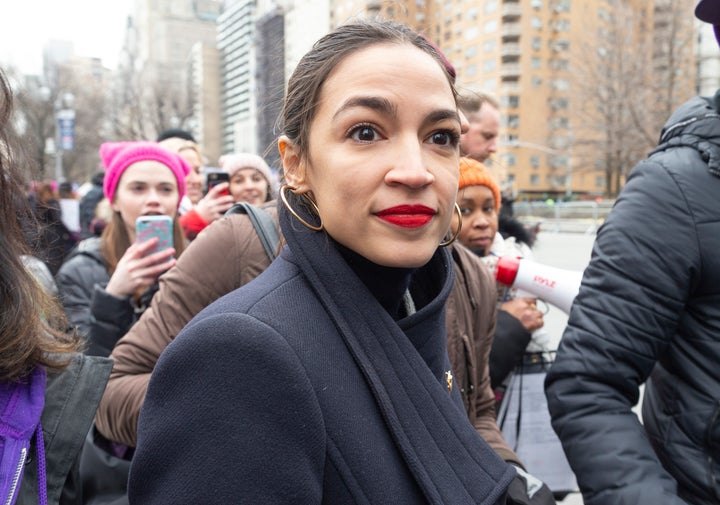
Speaking at an event on Monday, Congresswoman Alexandria Ocasio-Cortez warned of bias in machine learning. “Alexandria Ocasio-Cortez... claims that algorithms, which are driven by math, are racist”, sneered Ryan Saavedra, a reporter for the Daily Wire, on Twitter.
She’s right, though. Machine learning – the process by which computers can learn and improve the way they make decisions – is anything but perfect, and it’s high time we realise it.
If a kid comes back from school and explains to you that 2+2=5, there are two possible explanations. It’s possible the kid is defective and should be replaced. Or, more likely, there is a mistake in the way that child has been taught. Either the textbooks are wrong, or the person teaching them is wrong.
The way we teach machines isn’t dissimilar. Machines learn from data – the textbooks in the example above – and if that data is biased, or incomplete, or just plain wrong, the machine will likely inherit the biases, the gaps in knowledge and the mistakes.
“Garbage in, garbage out,” has been a touchstone of computer programming for decades. Cathy O’Neil, Zeynep Tufekci and Demos’s own Jamie Bartlett are among dozens of individuals and organisations who’ve been talking about the perils of machine learning for years.
If you put the wrong numbers into your calculator, you’ll get the wrong answer at the end. If you only train your soap dispenser on white hands, don’t be surprised when it fails everyone else.
But Saavedra’s sneering shows how easy it is to forget this. It’s just “math”, right? Computers, the argument goes, are just machines. How could the ones and the zeroes possibly be racist?
Through literature and film, we have told and retold the story of the journey towards techno-perfection. Running the universe in Iain M. Banks’ Culture novels is handed over to the hyperintelligent machines called Minds. Terminator’s Skynet was given control over US military hardware precisely because it was felt a computer wouldn’t make mistakes.
“Let me put it this way,” explains the villain of 2001: A Space Odyssey, HAL-9000, an artificial intelligence: “The 9000 series is the most reliable computer ever made. No 9000 computer has ever made a mistake or distorted information. We are all, by any practical definition of the words, fool-proof and incapable of error.”
This might have made sense in the past. It was pretty obvious when a machine wasn’t doing what it was supposed to. A car should start, and if it doesn’t it’s broken. An alarm clock should wake you up, and if it doesn’t go off, it’s broken. But when dealing with the most complicated tasks we can set computers, there are no right answers. At their core, algorithms are making as good a guess they can, based on the information they’ve been given and the task they have been set. This is going to require a step change in how we think about tech-driven decision-making. It won’t be right or wrong, working or broken, but just probably (hopefully) good.
If a machine-learning algorithm, for instance, pushes one news article higher than another in my Facebook feed or my Google results, it is impossible for me personally to tell whether it’s made the ‘right’ decision. If my sat nav recommends one route over another, I hope it’s ‘right’, but I am similarly blind to its decision-making process. Perhaps it took a new route to avoid traffic? Perhaps there are roadworks I didn’t know about. I have no idea. The decision-making process is utterly opaque, and therefore beyond serious evaluation. But I’ll follow it anyway.
This maps poorly to sci-fi’s ‘infallible machines’, and our trust in these systems ought to reflect that. Blindly following a sat nav that’s taking you into a traffic jam (or to Carpi instead of Capri) is not the end of the world, but when decisions on policing, disaster relief and healthcare are being made by an AI, it’s vital we remember that, at the end of the day, they are still just good guesses made on whatever data we fed the machine.
There will be frustration, no doubt. Governments and citizens are increasingly desperately looking for explanations for why technology is doing the things it is. Unfortunately, it feels like these questions are going to become harder to answer, not easier. We may have to submit to not knowing, or look for new ways of evaluating and accrediting technology that isn’t just based on a transparent code base. With this will come a change in expectations, too: we will have to accept that HAL 9000′s pretences to perfection will remain in the pages of science fiction, and that an algorithm really can be racist.
Alex Krasodomski-Jones is director of the Centre for Analysis of Social Media at Demos